Table of Contents
Examples of AI Bias in Healthcare: Understanding the Challenges and Solutions
Artificial intelligence (AI) is revolutionizing healthcare, offering unprecedented advancements in diagnosis, treatment, and patient care. However, alongside its potential, AI bias in healthcare is a critical issue that threatens equitable treatment and optimal outcomes for all. This bias, if left unchecked, disproportionately affects marginalized and underrepresented communities, undermining the promise of AI as a tool for progress.
In this article, we explore real-world examples of AI bias in healthcare, their impacts, and actionable strategies to address these challenges effectively.
Understanding the Basics of AI Bias in Healthcare
To appreciate the gravity of AI bias in healthcare, it’s essential to grasp its foundations.
What Is AI Bias?
AI bias occurs when algorithms produce skewed or unfair outcomes due to unrepresentative training data, flawed design, or embedded assumptions. In healthcare, such biases can result in disparities in diagnosis, treatment, and access to care.
Why Does It Matter?
AI has seen remarkable growth in healthcare, from diagnosing diseases to personalizing treatment plans. However, as reliance on AI increases, so does the need to ensure its fairness and accuracy. Bias in these systems can lead to life-altering consequences, particularly for already underserved populations.
Common Challenges
A major challenge lies in the lack of diversity in the data used to train AI systems. Without representation from all demographics, these systems fail to account for the unique needs of different populations, resulting in inequitable care. Additionally, the misconception that AI is inherently objective further complicates its implementation.
Five Real-world Examples of AI Bias in Healthcare
Let’s dive into five impactful examples of AI Bias and how they reveal the urgency of addressing this issue:
1. Bias in Diagnostic Algorithms
In 2019, researchers discovered that a diagnostic algorithm used in U.S. hospitals prioritized white patients over black patients. The algorithm measured healthcare needs based on past healthcare expenditures, which inadvertently disadvantaged black patients who historically face unequal access to care.
- Impact: This bias led to delayed diagnoses and inadequate treatments for black patients, exacerbating health disparities.
- Takeaway: Algorithms must consider actual healthcare needs rather than relying on biased proxies like past expenditures.
2. Racial Disparities in Treatment Recommendations
AI’s potential to personalize treatments is undermined when it fails to represent diverse populations. For example, certain algorithms overlook the higher prevalence of heart disease in black and Latinx communities due to underrepresentation in training data.
- Impact: Misdiagnoses and treatment delays disproportionately affect these populations, particularly in underserved areas like rural Mississippi.
- Takeaway: Incorporating diverse datasets can ensure treatment recommendations are inclusive and equitable.
3. Gender Bias in Screening Tools
AI tools used to screen for liver disease often display gender bias. A study from University College London found that AI models were twice as likely to miss liver disease in women compared to men because the training data skewed heavily toward male patients.
- Impact: Women face delayed diagnoses, leading to more severe health outcomes and higher treatment costs.
- Takeaway: AI systems must be rigorously evaluated for gender inclusivity before deployment.
4. Predictive Analytics and Resource Allocation
Predictive analytics, used to forecast patient outcomes and allocate healthcare resources, can be prone to bias if built on incomplete datasets. For example, models predicting hospital readmissions often fail to account for socioeconomic factors.
- Impact: Patients from low-income backgrounds may be inaccurately categorized as “low risk,” denying them essential preventive care.
- Takeaway: Socioeconomic data must be integrated to create fair and effective predictive models.
5. Ethical Concerns in AI-Powered Medical Devices
AI-driven medical devices hold immense promise but also pose ethical challenges. An international task force of bioethicists highlighted how poorly designed devices could perpetuate inequities in healthcare delivery.
- Impact: Misdiagnoses and inappropriate treatments erode trust in AI and risk harm to vulnerable populations.
- Takeaway: Human oversight and ethical guidelines are critical in the design and deployment of AI-powered devices.
Addressing AI Bias in Healthcare
The examples above highlight the urgent need to combat AI bias. Here’s how stakeholders can take proactive steps to ensure fair and effective AI systems:
- Employ Diverse Data Sets: Training AI on comprehensive and representative datasets reduces biases and improves accuracy.
- Ensure Human Oversight: Ethical oversight ensures algorithms make decisions aligned with human values.
- Conduct Bias Testing: Regular audits help identify and address biases in AI systems.
- Build Inclusive Teams: Diverse teams bring varied perspectives, reducing the risk of blind spots in algorithm design.
- Engage in Feedback Loops: Continuous monitoring and real-world testing help refine AI performance and ensure fairness.
The Way Forward
AI bias in healthcare is not merely a technical issue—it’s a societal challenge with real human consequences. Tackling this bias requires collaboration between developers, healthcare providers, policymakers, and communities. By committing to diversity, transparency, and ethical practices, we can build AI systems that enhance healthcare while ensuring equitable access and outcomes for all.
Key Takeaways
- Diagnosis and Treatment Biases: Algorithms often reflect existing inequities, leading to poor outcomes for marginalized groups.
- Data Representation Matters: Diverse datasets are essential for inclusive and effective AI systems.
- Human Oversight Is Key: Ethical standards and monitoring are vital to mitigate risks and build trust.
- Proactive Strategies: Regular audits, inclusive development, and feedback loops are necessary to reduce bias.
Reflection
As AI systems become increasingly integral to healthcare, their fairness and transparency will define their impact. How do you see the fight against AI bias in healthcare shaping the future of medicine? Share your thoughts and ideas in the comments below.
Citations
Related Post
AI Insight
Gain valuable AI insights with expert analysis, practical guides, and real-world applications. Explore trends, breakthroughs, and thought-provoking ideas to stay ahead in the evolving world of artificial intelligence.
AI Tools - Coming Soon
Discover the ultimate collection of AI tools to enhance your productivity, creativity, and efficiency. Explore cutting-edge apps and platforms for beginners and professionals, tailored to meet your needs across various industries.
Podcasts - Coming Soon
Explore the best AI podcasts featuring expert interviews, deep dives into trends, and real-world applications of artificial intelligence. Stay updated, inspired, and informed with engaging discussions on all things AI.
Related Posts
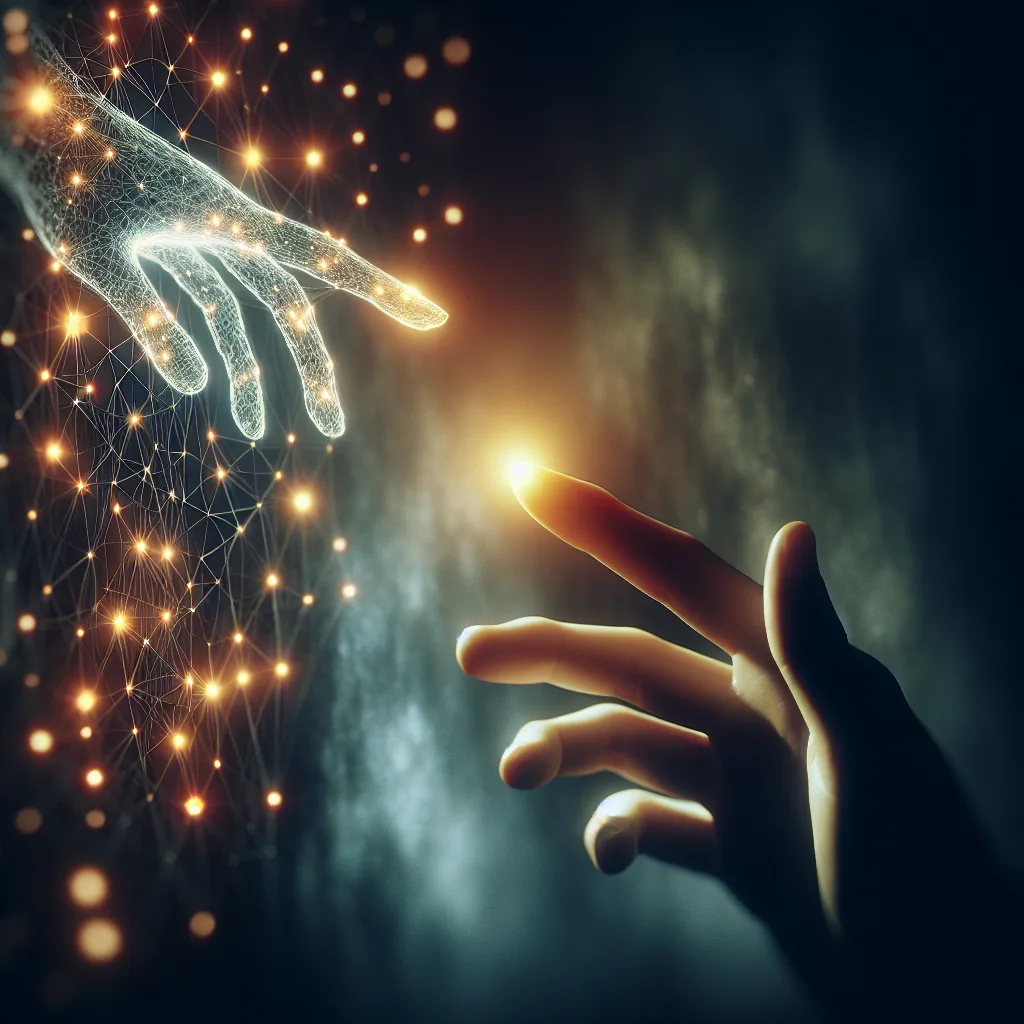
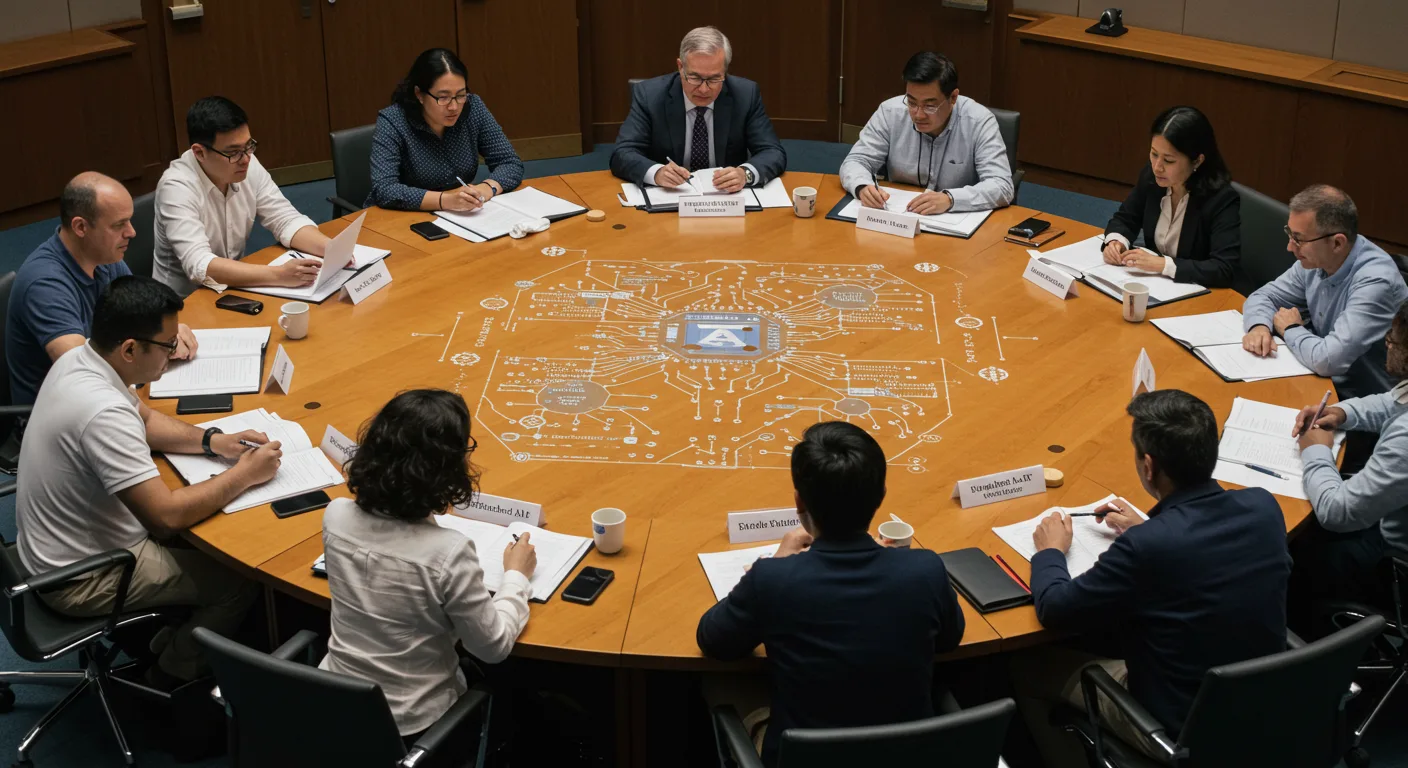

AI Ethics: Exploring Future Trends for a Responsible World

Unlocking the Brain: Understanding Neural Networks for Everyone
